Low Dimensional Motor Skill Learning Using Coactivation
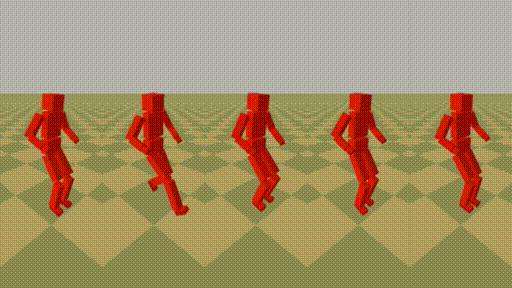
We propose an approach for motor skill learning in highly articulated characters by exploring low-dimensional joint coactivation spaces. Analysis of human motion reveals that many tasks have lower dimensionality than the character's full degrees of freedom (DOFs), with joints often moving in synchrony. We exploit this redundancy by extracting task-specific joint coactivations from human motion data, capturing synchronized patterns that reduce the control space. Using deep reinforcement learning, we train controllers to excite these coactivations, enabling humanlike control with only a few dimensions. We demonstrate this on various motor tasks, showing flexibility with different reward functions. By learning a 10-dimensional controller for a 28 DOF character, we achieve high-fidelity locomotion even with sparse rewards.
Highlights Video and Presentation
Publication
A. Ranganath P. Xu, I. Karamouzas, and V Zordan, “Low Dimensional Motor Skill Learning Using Coactivation”, in Motion, Interaction and Games, 2019, Newcastle, UK, Oct 2019. [PDF]