Motor Babble: Morphology Driven Coordinated Control of Articulated Characters
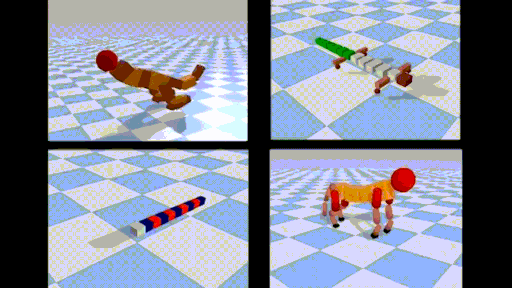
Locomotion in humans and animals is highly coordinated. Learning similar coordinated locomotion in virtual characters without reference motion data is challenging due to high dimensionality and redundancy. This paper presents a method for learning locomotion in a low-dimensional latent space defining joint coordination. We introduce "motor babble," where a character interacts with its environment through uncoordinated motor excitations. This generates a motion corpus from which a manifold latent space is extracted. Dimensions of this manifold represent character synergies. Using reinforcement learning, we train the character to locomote in this latent space by selecting appropriate dimensions and learning the corresponding policy.
Highlights Video
Publication
A. Ranganath A. Biswas, I. Karamouzas, and V Zordan, “Motor Babble: Morphology Driven Coordinated Control of Articulated Characters”, in Motion, Interaction and Games, 2021, Lausanne, Switzerland, Nov 2021. [PDF]